Thousands of people using the London Underground had their movements, behavior, and body language watched by AI surveillance software designed to see if they were committing crimes or were in unsafe situations, new documents obtained by WIRED reveal. The machine-learning software was combined with live CCTV footage to try to detect aggressive behavior and guns or knives being brandished, as well as looking for people falling onto Tube tracks or dodging fares.
From October 2022 until the end of September 2023, Transport for London (TfL), which operates the city’s Tube and bus network, tested 11 algorithms to monitor people passing through Willesden Green Tube station, in the northwest of the city. The proof of concept trial is the first time the transport body has combined AI and live video footage to generate alerts that are sent to frontline staff. More than 44,000 alerts were issued during the test, with 19,000 being delivered to station staff in real time.
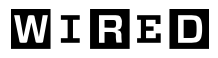
Documents sent to WIRED in response to a Freedom of Information Act request detail how TfL used a wide range of computer vision algorithms to track people’s behavior while they were at the station. It is the first time the full details of the trial have been reported, and it follows TfL saying, in December, that it will expand its use of AI to detect fare dodging to more stations across the British capital.
In the trial at Willesden Green—a station that had 25,000 visitors per day before the COVID-19 pandemic—the AI system was set up to detect potential safety incidents to allow staff to help people in need, but it also targeted criminal and antisocial behavior. Three documents provided to WIRED detail how AI models were used to detect wheelchairs, prams, vaping, people accessing unauthorized areas, or putting themselves in danger by getting close to the edge of the train platforms.
The documents, which are partially redacted, also show how the AI made errors during the trial, such as flagging children who were following their parents through ticket barriers as potential fare dodgers, or not being able to tell the difference between a folding bike and a non-folding bike. Police officers also assisted the trial by holding a machete and a gun in the view of CCTV cameras, while the station was closed, to help the system better detect weapons.
Privacy experts who reviewed the documents question the accuracy of object detection algorithms. They also say it is not clear how many people knew about the trial, and warn that such surveillance systems could easily be expanded in the future to include more sophisticated detection systems or face recognition software that attempts to identify specific individuals. “While this trial did not involve facial recognition, the use of AI in a public space to identify behaviors, analyze body language, and infer protected characteristics raises many of the same scientific, ethical, legal, and societal questions raised by facial recognition technologies,” says Michael Birtwistle, associate director at the independent research institute the Ada Lovelace Institute.
In response to WIRED’s Freedom of Information request, the TfL says it used existing CCTV images, AI algorithms, and “numerous detection models” to detect patterns of behavior. “By providing station staff with insights and notifications on customer movement and behaviour they will hopefully be able to respond to any situations more quickly,” the response says. It also says the trial has provided insight into fare evasion that will “assist us in our future approaches and interventions,” and the data gathered is in line with its data policies.
In a statement sent after publication of this article, Mandy McGregor, TfL’s head of policy and community safety, says the trial results are continuing to be analyzed and adds, “there was no evidence of bias” in the data collected from the trial. During the trial, McGregor says, there were no signs in place at the station that mentioned the tests of AI surveillance tools.
“We are currently considering the design and scope of a second phase of the trial. No other decisions have been taken about expanding the use of this technology, either to further stations or adding capability.” McGregor says. “Any wider roll out of the technology beyond a pilot would be dependent on a full consultation with local communities and other relevant stakeholders, including experts in the field.”
Computer vision systems, such as those used in the test, work by trying to detect objects and people in images and videos. During the London trial, algorithms trained to detect certain behaviors or movements were combined with images from the Underground station’s 20-year-old CCTV cameras—analyzing imagery every tenth of a second. When the system detected one of 11 behaviors or events identified as problematic, it would issue an alert to station staff’s iPads or a computer. TfL staff received 19,000 alerts to potentially act on and a further 25,000 kept for analytics purposes, the documents say.
The categories the system tried to identify were: crowd movement, unauthorized access, safeguarding, mobility assistance, crime and antisocial behavior, person on the tracks, injured or unwell people, hazards such as litter or wet floors, unattended items, stranded customers, and fare evasion. Each has multiple subcategories.
Daniel Leufer, a senior policy analyst at digital rights group Access Now, says whenever he sees any system doing this kind of monitoring, the first thing he looks for is whether it is attempting to pick out aggression or crime. “Cameras will do this by identifying the body language and behavior,” he says. “What kind of a data set are you going to have to train something on that?”
The TfL report on the trial says it “wanted to include acts of aggression” but found it was “unable to successfully detect” them. It adds that there was a lack of training data—other reasons for not including acts of aggression were blacked out. Instead, the system issued an alert when someone raised their arms, described as a “common behaviour linked to acts of aggression” in the documents.
“The training data is always insufficient because these things are arguably too complex and nuanced to be captured properly in data sets with the necessary nuances,” Leufer says, noting it is positive that TfL acknowledged it did not have enough training data. “I’m extremely skeptical about whether machine-learning systems can be used to reliably detect aggression in a way that isn’t simply replicating existing societal biases about what type of behavior is acceptable in public spaces.” There were a total of 66 alerts for aggressive behavior, including testing data, according to the documents WIRED received.
Madeleine Stone, a senior advocacy officer at privacy-focused group Big Brother Watch, says that many Tube travelers will be “disturbed” to learn that authorities subjected people to AI-powered surveillance. Stone says that using an algorithm to determine whether someone is “aggressive” is “deeply flawed” and points out that the UK’s data regulator has warned against using emotion analysis technologies.
Staff at the transportation body ran “extensive simulations” at Willesden Green station during the trial to gather more training data, the documents say. These included members of staff falling on the floor, and some of these tests happened when the station was closed. “You will see the BTP [British Transport Police] officer holding a machete and handgun in different locations within the station,” one caption in the documents state, although the images are redacted. During the trial, the files say, there were no alerts for weapons incidents at the station.
The most alerts were issued for people potentially avoiding paying for their journeys by jumping over or crawling under closed fare gates, pushing gates open, walking through open gates, or tailgating someone who paid. Fare dodging costs up to £130 million per year, TfL says, and there were 26,000 fare evasion alerts during the trial.
During all of the tests, images of people’s faces were blurred and data was kept for a maximum of 14 days. However, six months into the trial, the TfL decided to unblur the images of faces when people were suspected of not paying, and it kept that data for longer. It was originally planned, the documents say, for staff to respond to the fare dodging alerts. “However, due to the large number of daily alerts (in some days over 300) and the high accuracy in detections, we configured the system to auto-acknowledge the alerts,” the documents say.
Birtwistle, from the Ada Lovelace Institute, says that people expect “robust oversight and governance” when technologies like these are put in place. “If these technologies are going to be used, they should only be used with public trust, consent and support,” Birtwistle says.
A large part of the trial was aimed at helping staff understand what was happening at the station and respond to incidents. The 59 wheelchair alerts allowed staff at Willesden Green station, which does not have access facilities for wheelchairs, to “provide the necessary care and assistance,” the files say. Meanwhile, there were almost 2,200 alerts for people going beyond yellow safety lines, 39 for people leaning over the edge of the track, and almost 2,000 alerts for people sitting on a bench for extended periods.
“Throughout the PoC we have seen a huge increase in the number of public announcements made by staff, reminding customers to step away from the yellow line,” the documents say. They also say the system generated alerts for “rough sleepers and beggars” at the station’s entrances and claim this allowed staff to “remotely monitor the situation and provide the necessary care and assistance.” TfL states that the system was trialed to try to help it improve the quality of staffing at its stations and make it safer for passengers.
The files do not contain any analysis of how accurate the AI detection system is; however, at various points, the detection had to be adjusted. “Object detection and behavior detection are generally quite fragile and are not foolproof,” Leufer, of Access Nows, says. In one instance, the system created alerts saying people were in an unauthorized area when in reality train drivers were leaving the train. Sunlight shining onto the camera also made them less effective, the documents say.
The tests also aimed to see if unfolded or nonfolding bikes and escooters—which are largely not allowed on the transport network—could be detected by AI. “The Al could not differentiate between an unfolded bike and normal bike and an e-scooter and children’s scooter,” the documents state. The fare dodging model also flagged children. “During school travelling hours, we would see a spike in parent and child tailgating alerts,” the documents say. The system was adjusted to not flag people “whose height was below the gate.”
In recent years, the use of AI in public spaces to detect people’s behaviors, movements, or identities has increased—often through the guise of smart city approaches. In July last year, reports revealed that several New York City subway stations are using AI to track fare evasion. In London, TfL said in December it would expand its fare evasion trials to other stations, although the status of those experiments is unknown
Many of these systems are being developed in situations where there is a lack of specific laws governing their use, with warnings of a regulatory vacuum in the UK. “Normalizing AI-powered monitoring at transport hubs is a slippery slope towards a surveillance state, and must be subject to greater transparency and public consultation,” says Stone from Big Brother Watch.
Leufer warns that once these systems start to be used more widely, they can always be upgraded. “Once the infrastructure is there, it’s absolutely trivial to update what it does,” he says. “It’s really worrying, what could be added to these things. The slippery slope is very slippery.”
This story originally appeared on wired.com.